Real-world Applications of Graph Neural Networks
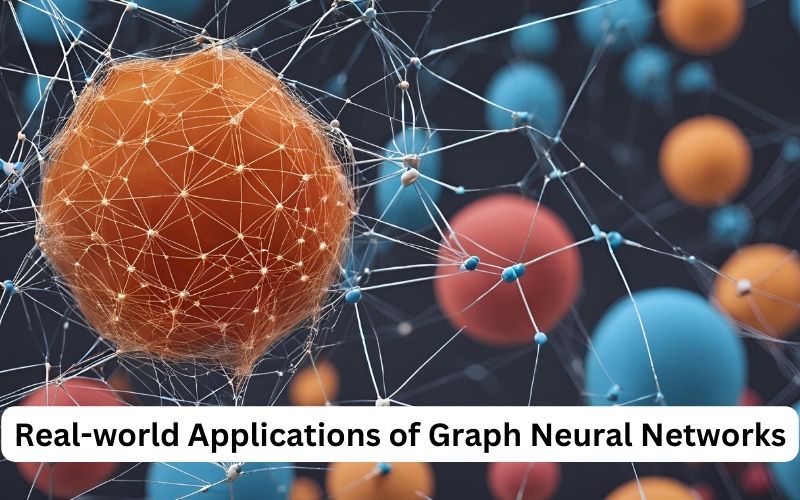
Graph Neural Networks (GNNs) are an impactful class of machine learning models designed to work with graph-structured data. They have gained massive attention due to their ability to capture relationships and dependencies between entities in a graph. GNNs are transforming various fields from social to biological networks by providing deeper insights and more accurate predictions. For those interested in mastering this cutting-edge technology, enrolling in a data scientist course in Hyderabad can be an excellent way to gain the necessary skills and knowledge.
Understanding Graph Neural Networks
Graphs are a natural way to represent data where relationships between entities matter. Examples include social networks, where nodes represent individuals and edges represent connections; molecular structures, where nodes represent atoms and edges represent bonds; and transportation networks, where nodes represent locations and edges represent routes. GNNs leverage the structure of graphs to perform tasks, viz. node classification, link prediction, and graph classification.
By taking a data science course, students can learn the foundational concepts of GNNs, including graph theory, neural network architectures, and the algorithms used to process and analyze graph data. These courses provide the theoretical background and practical skills needed to apply GNNs to real-world problems.
Social Network Analysis
One of the most prominent applications of GNNs is in social network analysis. Social networks such as Facebook as well as Twitter can be represented as graphs where users are nodes and their connections are edges. GNNs can analyze these networks to uncover hidden patterns and insights. For example, they can be used to identify influential users, detect communities, and predict future connections.
Enrolling in a data scientist course in Hyderabad allows individuals to gain hands-on experience with these applications. Hyderabad’s thriving tech scene offers ample opportunities for students to take up real-world projects and collaborate with industry experts, providing a practical understanding of how GNNs can be applied to social network analysis.
Drug Discovery and Molecular Biology
In the field of drug discovery, GNNs are making significant strides by enabling more accurate predictions of molecular properties and interactions. Molecules can be represented as graphs, with atoms as nodes and chemical bonds as edges. GNNs can predict the biological activity of new compounds, identify potential drug candidates, and understand the interactions between different molecules.
A data science course covers the principles of applying GNNs to biological networks, including techniques for graph representation and the use of specialized GNN architectures. This knowledge is crucial for researchers and professionals working in drug discovery and molecular biology, as it allows them to leverage GNNs to accelerate the development of new therapies and treatments.
Recommendation Systems
Recommendation systems are another area where GNNs are proving to be highly effective. By representing users and items as nodes in a graph and interactions between them as edges, GNNs can provide personalized recommendations with high accuracy. This approach is particularly useful for platforms like e-commerce sites and streaming services, where understanding user preferences and item relationships is key to delivering relevant recommendations.
Financial Fraud Detection
Detecting fraudulent activities is a critical challenge in the financial sector. Financial transactions can be represented as graphs, where nodes represent entities such as accounts and transactions and edges represent relationships between them. GNNs can analyze these graphs to detect suspicious patterns and anomalies that may indicate fraud.
A data science course provides the necessary training to apply GNNs to financial fraud detection. Students learn how to preprocess financial data, construct graphs, and implement GNN models to identify fraudulent activities. This expertise is invaluable for finance, cybersecurity, and risk management professionals.
Traffic and Transportation Networks
GNNs are also being used to optimize traffic and transportation networks. By modeling cities as graphs, with intersections as nodes and roads as edges, GNNs can predict traffic flow, optimize routing, and improve transportation infrastructure. This application is particularly relevant for smart cities aiming to enhance urban mobility and reduce congestion.
A data scientist course in Hyderabad offers opportunities to work on projects related to traffic optimization and smart city initiatives. Hyderabad’s expanding urban landscape provides a rich environment for studying and applying GNNs to real-world transportation problems, preparing students for urban planning and intelligent transportation systems careers.
Conclusion
Graph Neural Networks are revolutionizing various fields by providing powerful tools to analyze and interpret graph-structured data. From social network analysis and drug discovery to recommendation systems and traffic optimization, the applications of GNNs are vast and impactful. For those looking to master this technology, enrolling in a data science course offers a comprehensive education in the principles and practices of GNNs. These courses equip students with the knowledge needed to apply GNNs to real-world problems, opening up exciting career opportunities in a rapidly transforming field.
ExcelR – Data Science, Data Analytics and Business Analyst Course Training in Hyderabad
Address: Cyber Towers, PHASE-2, 5th Floor, Quadrant-2, HITEC City, Hyderabad, Telangana 500081
Phone: 096321 56744